Digital Maintenance Solutions in the Renewable Energy Sector: Implementation Barriers and Value Drivers
1. Introduction
Over the past two decades, the availability and ubiquity of digital infrastructures contributed to the development of new technologies, producing a strong disruptive effect on industrial life in general and the value chain, in particular (Kuhn & Lucke, 2021). To remain competitive and increase their market share, many companies across industries leverage Industry 4.0 technologies, including cloud computing, Big Data, and artificial intelligence (AI) (Gorenstein & Kalech, 2022). The implementation of digital technologies enables firms to improve and optimise energy management and generation processes. It has also facilitated the emergence of self-regulated, learning-oriented, intelligent maintenance focused on improving the economic and technical effectiveness of companies’ maintenance measures (Es-sakali et al., 2022).
While the new digitised context implies changes in how organisations run their business operations, too little is known about the maintenance digital transformation phenomenon in the renewable energy sector (Mrazovac et al., 2020). This report attempts to provide new insights into this research problem by focusing on how digital maintenance solutions could contribute to the performance of renewable energy firms and what barriers could prevent them from introducing smart maintenance measures.
2. Renewable Energy Sector
The renewable energy sector produces energy from resources that can be renewed within a human lifetime and include wind, sunlight, waves, geothermal heat, and wood residues (Sa’ad et al., 2022). The use of renewables has grown significantly in recent years and accounted for around 14% of the world’s energy consumption in 2019 (The World Bank, 2022; IEA, 2019). In the European Union, this rate is even higher as renewable energy represented more than 22% of the total amount of energy consumed in 2020 (Eurostat, 2022; OECD, 2022). The growing demand for renewable energy is predominantly explained by several factors. First, renewables are commonly viewed as an important step in dealing with the problem of climate change and its consequences (Deloitte, 2022).
Figure 1: Global Carbon Dioxide Emissions from Fossil Fuels, 1950-2020 (in Billion Metric Tons)
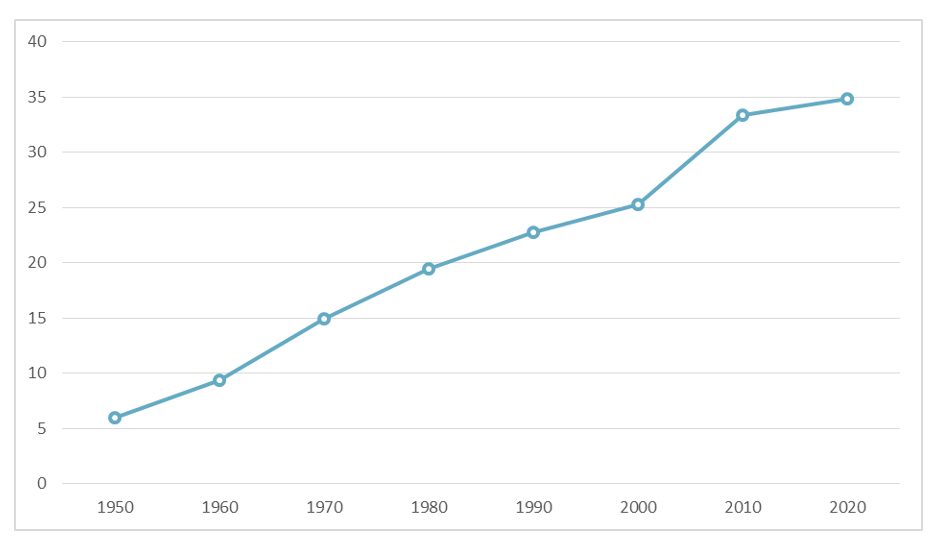
Note. Based on Tiseo (2022, n.p.)
Second, growing populations and urbanisation put significant pressure on the global economy, which results in increased reliance on fossil fuel sources for energy generation (IEA, 2022). However, technological shortcomings, antiquated infrastructure, and the growing cost of energy production using conventional sources do not allow for meeting the continuously growing demand for electricity in many areas of the world (Deloitte, 2022). In turn, the decreasing cost of renewable technologies makes the production of green energy a highly attractive option for those countries willing to reduce their reliance on fossil fuel energy sources and strengthen their energy security (Es-sakali et al., 2022).
Figure 2: Renewable Energy Capacity Worldwide (in Gigawatts)
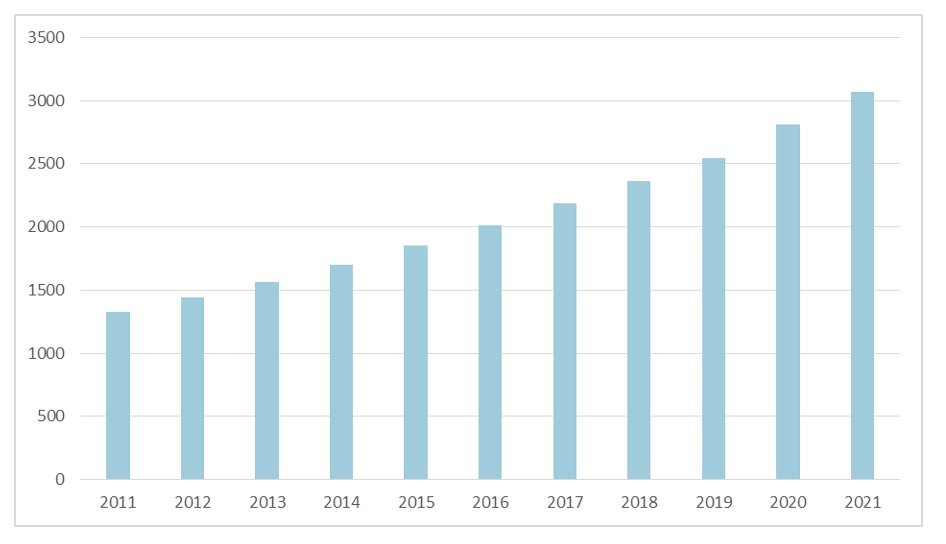
Note. Based on Jaganmohan (2022, n.p.)
Despite the momentum the renewable energy sector has recently gained, as well as the growing availability of leading technologies, around 70% of all digital transformations fail (Garcia, 2022). Moreover, the reliance of renewable energy organisations on decentralised energy production creates additional challenges to the effectiveness of the whole system (Gorenstein & Kalech, 2022). That is why the role of digital maintenance, which emphasises increased efficiency levels achieved through automated data collection, advanced analysis, and structured decision-making, in the development of the renewable energy sector is crucial (van Dinter et al., 2022).
3. The Need for Digital Maintenance Solutions
The reliability and availability of equipment, especially in asset-intensive industries, such as renewable energy production, have always been one of the biggest topics (Es-sakali et al., 2022). Traditional approaches to maintenance operations involve human intervention, which is associated with several drawbacks. Humans tend to make mistakes, as well as less precise reasoning, resulting in the poor calculation accuracy and increased response times (Torrent-Sellens et al., 2022). Moreover, traditional maintenance processes involve heavy paperwork due to human-to-machine communication, which not only raises maintenance costs but also makes maintenance management less efficient (Magistretti et al., 2021). Considering the level of competition in the renewable energy industry, effectively managing both planned and unplanned outages while increasing maintenance-labour productivity is key to success.
As previously noted, the renewable energy industry is characterised by decentralised energy production. Therefore, an energy-producing company’s ability to provide effective and prompt maintenance services to its assets in a geographically remote location is limited. Historically, providing high-quality maintenance services in such remote locations was not cost-efficient and required significant financial and time resources (Sa’ad et al., 2022). Additionally, the effectiveness and quality of maintenance activities relied heavily on the consistent availability and accuracy of the asset information (Kuhn & Lucke, 2021). Considering the limitations of the traditional approach, maintenance was reactive in most cases, as maintenance personnel did not have precise and in-advance knowledge and understanding of how the asset performed (Kryukov et al., 2022). As a result, repairs were triggered by the breakdown, leading to downtimes that halted the productivity and operation of energy-producing organisations.
Digital maintenance can be defined as “learning-oriented, self-regulated, intelligence maintenance with the aim of maximising the technical and economic effectiveness of maintenance measures, taking into account the respective existing production system, through the use of digital applications” (Mrazovac et al., 2020, p.1). The emergence and development of the aforementioned Industry 4.0 technologies (e.g., Big Data, AI, robotics, simulation, and cloud computing) have enabled smart maintenance systems to learn about the assets, predict equipment malfunctions, and provide the required care in the form of automatically generated reports and alerts (Adamides & Karacapilidis, 2020). The digitisation of maintenance procedures and processes allows for overcoming the limits of geographical boundaries and enables companies to take a more proactive approach to maintenance (van Dinter et al., 2022). Given the forecasted size of the renewable energy market, the implementation of digital maintenance solutions will determine the extent to which companies can remain competitive.
Figure 3: Clean Energy Market Size (in $ Billion)
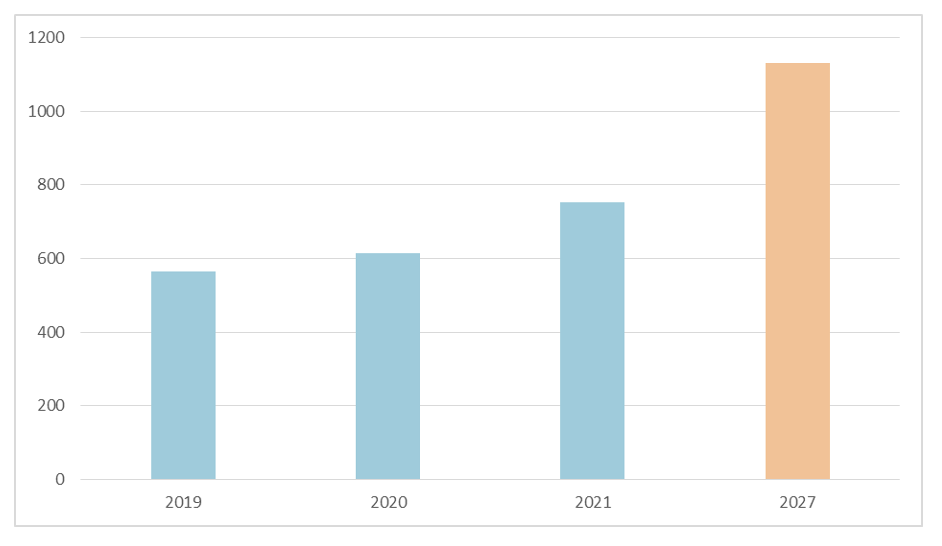
Note. Based on IEA (2019, n.p.), Jaganmohan (2021, n.p.)
At the same time, using advanced-analytics capabilities and digital instruments does not automatically translate into increased productivity and a higher rate of prevented equipment failures (Martínez-Caro et al., 2020). For instance, the training phase requires tuning and adjusting the model architecture and its parameters. Hence, the lack of experience or knowledge can prevent the AI from performing as intended, posing a threat to the effectiveness and efficiency of the digital maintenance system as a whole (Marcon et al., 2022).
4. Implementation Barriers
The literature that addresses the key barriers to the implementation of digital maintenance solutions is fragmented and scarce. Many previous studies among those available approach this issue from the perspective of the technology acceptance theory (Gorenstein & Kalech, 2022). In essence, the existing body of knowledge that relies on this theoretical framework distinguishes between technological, security, and organisational barriers. For example, Oesterreich and Teuteberg (2016) reported that companies had to ensure that their strategies were aligned with the different systems and processes that had to be automated to successfully digitise maintenance. In turn, the lack of expertise and experience was noted by Saihi et al. (2022) as the key technological aspect that prevented business entities from introducing digital maintenance solutions. This factor could negatively affect the ability to select the right automation instruments and technologies and recognise the right automation characteristics, leading to the suboptimal performance of the maintenance system (Adamides & Karacapilidis, 2020).
Table 1: Main Barriers to the Implementation of Digital Maintenance
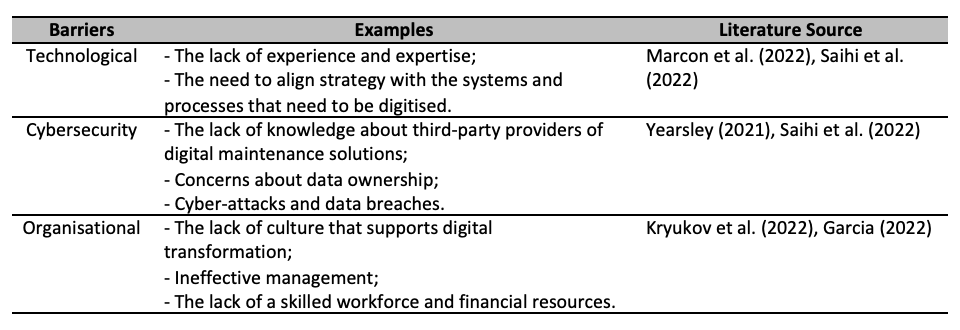
The lack of a skilled workforce, digital culture, and effective management is also commonly attributed to the major barriers to the implementation of Industry 4.0 technologies and digital maintenance solutions (Kryukov et al., 2022). In large international firms with multi-layered hierarchies, coordinating digitisation actions across different organisational units becomes challenging due to inert decision-making (Es-sakali et al., 2022). The development of information and communication technologies makes it easier for large companies to organise the process of maintenance digitisation. Still, the success of this process relies heavily on the extent to which their organisational culture is conducive to the execution of a digital transformation strategy (Magistretti et al., 2021). Due to the same reason, large multinational corporations, including those operating in the green energy sector, lag behind smaller organisations in terms of having a strong and common culture that supports digital transformation (Garcia, 2022). As noted by Martínez-Caro et al. (2020), many large companies have a culture averse to experimentation and risk, which results in the lack of courage to introduce such a radical change as maintenance digital transformation.
Smaller organisations are more flexible in decision-making and strategy formulating and adjusting, which gives them an advantage over large firms when it comes to change management and the implementation of digital maintenance solutions (Vial, 2021). One could argue that the digitisation of a renewable energy firm’s maintenance system requires significant investments to deploy connected technologies and take advantage of predictive asset analytics. This means that smaller companies are less capable of achieving this goal due to limited financial resources as compared to large business entities (Mrazovac et al., 2020).
While this assumption has been accepted by previous researchers in the field, the phenomenon of Industry 4.0 suggests that this situation is about to change in the nearest future (Oesterreich & Teuteberg, 2016; Torrent-Sellens et al., 2022; Roffia & Mola, 2022). Open innovation has made it possible for smaller companies, which do not have sufficient financial resources to invest in digital transformation, to source ideas and technologies from external sources (Adamides & Karacapilidis, 2020). Still, taking advantage of open innovation and shifting to digital maintenance requires the availability of highly developed information infrastructure and enterprise resource planning, which cannot be acquired through open innovation.
As evident from the above discussion, maintenance digital transformation and the implementation of digital maintenance solutions do not rely solely on technologies, corporate culture, or financial resources (Marcon et al., 2022). Although the role of automation in carrying out maintenance activities is growing, they still cannot be performed without personnel, which is deemed indispensable in many industries, renewable energy production included (Sa’ad et al., 2022). Several previous studies in the field emphasised the contribution of the human factor to the success of maintenance digital transformation and the implementation of digital maintenance solutions (Kuhn & Lucke, 2021). For example, Kryukov et al. (2022) examined the readiness of a transport company for digital transformation and concluded that the successful transition from traditional to digital operations and processes required highly specialised knowledge, as well as distinctive competencies. Similarly, Adamides and Karacapilidis (2020) argued that the lack of necessary talent was one of the main factors that hampered digitisation and automation.
The above barrier paves the way for many other challenges to the implementation of digital maintenance solutions. The need for highly skilled, talented employees who not only facilitate digital transformation but can also follow new ways of working and thinking requires continuous training and development (Kuhn & Lucke, 2021). In addition, a company must create a favourable work environment where its employees could develop a positive attitude towards change in order to adapt quickly to digitised and automated maintenance activities and processes (Vial, 2021). Even if all these factors are considered, a lack of a clear business case, which justifies heavy investments in the underlying digital maintenance architecture in the renewable energy sector could be viewed as an important barrier to its implementation (Saihi et al., 2022).
Last but not least implementation barrier refers to the issue of cybersecurity. The COVID-19 pandemic and its impact on businesses’ value and supply chains could be viewed as a major factor that has propelled the digitisation and automation of maintenance activities (Yearsley, 2021). However, with the growing reliance on Industry 4.0 technologies, the pressure on digital safety and security continues to rise (Garcia, 2022). According to the UK government, around half of all businesses operating in the country reported cybersecurity breaches or attacks in 2020 (Yearsley, 2021). Cybersecurity concerns are exacerbated by the fact that companies have to rely on third-party providers when implementing digital maintenance solutions. The lack of knowledge about these providers adds to business entities’ concerns about data ownership while creating additional challenges with integrating data from multiple sources to enable Industry 4.0 maintenance applications (Saihi et al., 2022).
5. Value Drivers
The digitisation and automation of maintenance operations and procedures in the renewable energy sector are associated with multiple factors that can increase the worth of the products and services it produces (Gorenstein & Kalech, 2022). The implementation of these solutions implies a major shift from reactive to proactive decision-making, which can bring numerous organisational benefits, starting from reduced maintenance and inventory holding costs to increased productivity and forecasting accuracy (Es-sakali et al., 2022; van Dinter et al., 2022).
Predictive maintenance is one of the main benefits brought by Industry 4.0 technologies that enable companies to predict and optimise the work of their assets more effectively as compared to traditional approaches to maintenance (Es-sakali et al., 2022). In the renewable energy sector, assets, equipment, and machines are expected to operate continuously and consistently for several months or even years until as guaranteed by the supplier or manufacturer (van Dinter et al., 2022). With that being stated, industrial components do not always perform as expected. Moreover, no green energy producer has the human resources to inspect all the equipment every minute for signs of failure. While continuous monitoring and routine maintenance to a certain extent help companies control failures, their effectiveness in identifying a particular point in time when the asset or equipment will break is limited (Dinis et al., 2022). This limitation not only increases the amount of plant and asset downtimes but also makes it challenging for maintenance personnel to accurately forecast component or asset failures.
Table 2: Benefits of Maintenance Digitisation and Automation
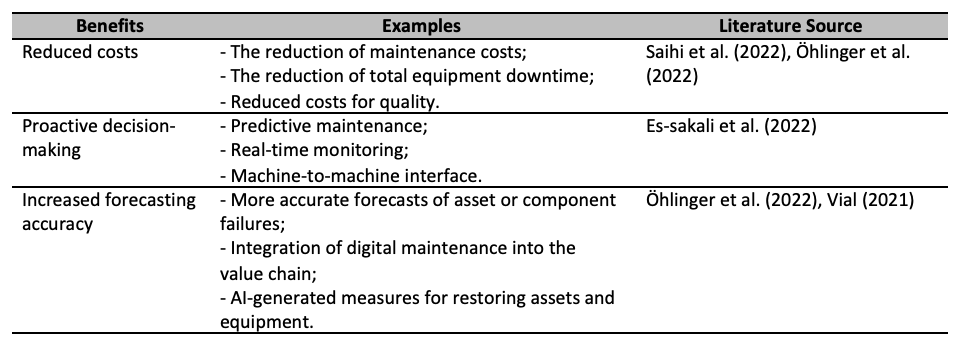
Digital maintenance solutions allow renewable energy-producing companies to overcome the aforementioned limitations and approach the process of maintenance more proactively. By using advanced process data analytics, monitoring data, predictive models, and learning algorithms, the predictive functionalities of these solutions can identify underperforming assets and make accurate predictions on upcoming failures (Karki et al., 2022). Add to that, the machine-to-machine interface makes it possible to monitor the performance of assets and equipment in real-time, which allows for getting a better understanding of their future performance (Gorenstein & Kalech, 2022). Predictive digital maintenance plays a crucial role in reducing maintenance costs and total equipment downtime. The existing literature indicates that business entities could use digital maintenance instruments to simulate different asset operations and, based on this simulation, find an optimal set point for a piece of equipment to balance its performance, efficiency, and reliability (Mrazovac et al., 2020). As a result, predictive and remote maintenance not only improves asset performance but also reduces maintenance costs, adding to a company’s competitiveness.
More effective and efficient asset utilisation can be viewed as an important value driver of digital maintenance, which could significantly reduce total equipment and asset downtime. As mentioned elsewhere in this report, the key difference between the renewable energy sector and the ‘conventional’ energy sector is that the former relies on decentralised energy production (Renewables 2021, 2021). This characteristic makes it challenging for market players to continuously monitor and control the work of assets and equipment in distant geographical areas and harsh or dangerous environments. Concurrently, digital maintenance solutions address this challenge, as they allow a maintenance worker to stream data about equipment vital stats in real-time with no need to visit the site in person (Saihi et al., 2022). This, in turn, significantly reduces the possibility of injury to and error by the operator. By using Industry 4.0 capabilities in maintenance, the operator can avoid alert fatigue since all the work monitoring a large volume of machinery and data is delegated to AI-powered maintenance (Martínez-Caro et al., 2020).
Forecast accuracy is another value driver that justifies the implementation of digital maintenance solutions in the renewable energy industry. The author has already emphasised the significance of predictive maintenance by arguing that the integration of AI and other Industry 4.0 technologies into the maintenance framework could facilitate a major shift from reactive maintenance to proactive maintenance (Vial, 2021). However, the role of digital maintenance is not limited to the ability to forecast the time of failure of individual components and assets. What is more important from a strategic perspective is the integration of digital maintenance into the entire value chain to achieve a higher level of connectivity between various organisational processes and activities (Karki et al., 2022). For example, by integrating AI into the value chain of technical service, renewable energy companies would be able to forecast the capacity requirements of their assets and equipment in the event of damage or failure. In other words, this further digitisation of the maintenance framework would allow for including the measures for restoring the equipment in the forecast rather than just predicting when it will fail (Öhlinger et al., 2022).
6. Conclusion
The role of maintenance in asset-intensive industries such as renewable energy production is hard to overestimate because it allows for ensuring that all the assets and equipment of a company required for the production of clean energy are operating as expected (Adamides & Karacapilidis, 2020). However, unlike the ‘conventional’ energy sector, the renewable energy industry relies on decentralised energy production, which poses unique challenges to maintenance effectiveness (Saihi et al., 2022). The transformation of maintenance from a typical analogue to a digital process, which is known as digitisation, is expected to revolutionise the way renewable energy organisations approach the process of maintenance (Karki et al., 2022). The machine-to-machine interface enables firms to monitor the performance of their assets and equipment in real-time and eliminates the possibility of human error. This could potentially translate into operational and financial benefits, as well as reduce equipment downtimes and maintenance costs (Vial, 2021).
While the contribution of digital maintenance solutions to the performance of clean energy companies is strong, the implementation of these solutions is associated with a range of challenges and barriers (Mrazovac et al., 2020). The lack of talent and organisational culture conducive to the execution of a digital transformation strategy, financial constraints, and cybersecurity issues, just to mention a few (Kuhn & Lucke, 2021; Saihi et al., 2022). However, the existing body of literature largely overlooks how business entities operating in the renewable energy sector could effectively respond to these challenges to integrate digital maintenance solutions into their value chain and enjoy their benefits (Saihi et al., 2022; Martínez-Caro et al., 2020).
References
Adamides, E., & Karacapilidis, N. (2020). Information technology for supporting the development and maintenance of open innovation capabilities. Journal of Innovation & Knowledge, 5(1), 29-38. https://doi.org/10.1016/j.jik.2018.07.001
Deloitte (2022). 2022 renewable energy industry outlook. Deloitte. https://www2.deloitte.com/us/en/pages/energy-and-resources/articles/renewable-energy-outlook.html
Dinis, D., Barbosa-Póvoa, A., & Teixeira, Â. P. (2022). Enhancing capacity planning through forecasting: An integrated tool for maintenance of complex product systems. International Journal of Forecasting, 38(1), 178-192. https://doi.org/10.1016/j.ijforecast.2021.05.003
Es-sakali, N., Cherkaoui, M., Mghazli, M., & Naimi, Z. (2022). Review of predictive maintenance algorithms applied to HVAC systems. Energy Reports, 8(1), 1003-1012. https://doi.org/10.1016/j.egyr.2022.07.130
Eurostat (2022). Renewable energy statistics. Eurostat. https://ec.europa.eu/eurostat/statistics-explained/index.php?title=Renewable_energy_statistics
Garcia, J. (2022). Common pitfalls in transformations: A conversation with Jon Garcia. McKinsey & Company. https://www.mckinsey.com/capabilities/transformation/our-insights/common-pitfalls-in-transformations-a-conversation-with-jon-garcia
Gorenstein, A., & Kalech, M. (2022). Predictive maintenance for critical infrastructure. Expert Systems with Applications, 210(1), 118413. https://doi.org/10.1016/j.eswa.2022.118413
IEA (2019). Global Energy Review 2019 – Renewables. IEA. https://www.iea.org/reports/global-energy-review-2019/renewables
IEA (2022). Renewable Electricity. IEA. https://www.iea.org/reports/renewable-electricity
Jaganmohan, M. (2021). Global renewable energy market size 2020-2027. Statista. https://www.statista.com/statistics/1094309/renewable-energy-market-size-global/
Jaganmohan, M. (2022). Global cumulative renewable capacity installed 2009-2021. Statista. https://www.statista.com/statistics/1094331/global-renewable-capacity-cumulative/
Karki, B., Basnet, S., Xiang, J., Montoya, J., & Porras, J. (2022). Digital maintenance and the functional blocks for sustainable asset maintenance service–A case study. Digital Business, 2(2), 100025. https://doi.org/10.1016/j.digbus.2022.100025
Kryukov, V., Shakhgeldyan, K., Kiykova, E., Kiykova, D., & Saychuk, D. (2022). Assessment of transport enterprise readiness for digital transformation. Transportation Research Procedia, 63(1), 2710-2718. https://doi.org/10.1016/j.trpro.2022.06.313
Kuhn, C., & Lucke, D. (2021). Supporting the digital transformation: a low-threshold approach for manufacturing related higher education and employee training. Procedia CIRP, 104(1), 647-652. https://doi.org/10.1016/j.procir.2021.11.109
Magistretti, S., Pham, C., & Dell’Era, C. (2021). Enlightening the dynamic capabilities of design thinking in fostering digital transformation. Industrial Marketing Management, 97(1), 59-70. https://doi.org/10.1016/j.indmarman.2021.06.014
Marcon, É., Le Dain, M., & Frank, A. (2022). Designing business models for Industry 4.0 technologies provision: Changes in business dimensions through digital transformation. Technological Forecasting and Social Change, 185(1), 122078. https://doi.org/10.1016/j.techfore.2022.122078
Martínez-Caro, E., Cegarra-Navarro, J., & Alfonso-Ruiz, F. (2020). Digital technologies and firm performance: The role of digital organisational culture. Technological Forecasting and Social Change, 154(1), 119962. https://doi.org/10.1016/j.techfore.2020.119962
Mrazovac, B., Hulea, M., Ilian, V., & Ristic, K. (2020). Smart Maintenance: The Evolution of Machine’s Response from Corrective to Predictive. Nexus Integra. https://nexusintegra.io/digital-transformation-renewable-energy/
OECD (2022). Renewable energy. OECD. https://data.oecd.org/energy/renewable-energy.htm
Oesterreich, T., & Teuteberg, F. (2016). Understanding the implications of digitisation and automation in the context of Industry 4.0: A triangulation approach and elements of a research agenda for the construction industry. Computers in Industry, 83(1), 121-139. https://doi.org/10.1016/j.compind.2016.09.006
Öhlinger, F., Greimel, L., Glawar, R., & Sihn, W. (2022). An approach for AI-based forecasting of maintenance orders for MRO scheduling. IFAC-PapersOnLine, 55(10), 2312-2317. https://doi.org/10.1016/j.ifacol.2022.10.053
Renewables 2021 (2021). Global Status Report. Ren21. https://www.ren21.net/wp-content/uploads/2019/05/GSR2021_Full_Report.pdf
Roffia, P., & Mola, L. (2022). Is COVID-19 enough? Which underestimated conditions characterise the adoption of complex information infrastructures in small and medium-sized enterprises. Journal of Business Research, 144(1), 1249-1255. https://doi.org/10.1016/j.jbusres.2021.12.083
Sa’ad, A., Nyoungue, A., & Hajej, Z. (2022). An integrated maintenance and power generation forecast by ANN approach based on availability maximization of a wind farm. Energy Reports, 8(1), 282-301. https://doi.org/10.1016/j.egyr.2022.06.120
Saihi, A., Ben-Daya, M., & As’ad, R. (2022). A Survey of the Underlying Success Factors of Maintenance Digital Transformation. IFAC-Papers on Line, 55(10), 2944-2949. https://doi.org/10.1016/j.ifacol.2022.10.179
Tiseo, I. (2022). Global historical CO2 emissions from fossil fuels and industry 1750-2020. Statista. https://www.statista.com/statistics/264699/worldwide-co2-emissions/
Torrent-Sellens, J., Díaz-Chao, Á., Miró-Pérez, A., & Sainz, J. (2022). Towards the Tyrell corporation? Digitisation, firm-size and productivity divergence in Spain. Journal of Innovation & Knowledge, 7(2), 100185. https://doi.org/10.1016/j.jik.2022.100185
van Dinter, R., Tekinerdogan, B., & Catal, C. (2022). Predictive maintenance using digital twins: A systematic literature review. Information and Software Technology, 151(1), 107008. https://doi.org/10.1016/j.infsof.2022.107008
Vial, G. (2021). Understanding digital transformation: A review and a research agenda. Managing Digital Transformation. https://www.sciencedirect.com/science/article/pii/S0263237322001219
World Bank, The (2022). Renewable energy consumption (% of total final energy consumption). The World Bank. https://data.worldbank.org/indicator/EG.FEC.RNEW.ZS
Yearsley, J. (2021). Accelerated digitalisation leave businesses susceptible to cyberattacks. Deloitte. https://www2.deloitte.com/uk/en/pages/consumer-business/articles/accelerated-digitalisation-leave-businesses-susceptible-to-cyberattacks.html